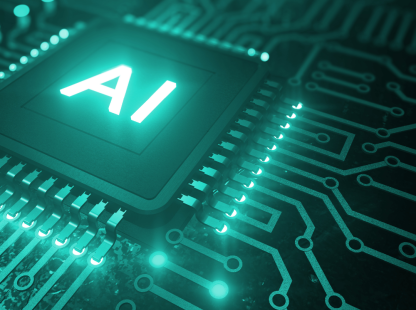
AI Core Competencies
The AI Core Standards are designed to equip all NJIT undergraduates with the essential knowledge and skills needed to navigate a world increasingly shaped by artificial intelligence.
The AI Core Competencies are designed to equip all NJIT undergraduates with the essential knowledge and skills needed to navigate a world increasingly shaped by artificial intelligence, particularly generative AI.
By graduation, every student will have developed a foundational understanding of AI's practical applications, ethical implications, and interdisciplinary significance.
These competencies ensure that graduates are not only literate in AI technologies but are also prepared to adapt to and lead in industries where AI is transforming workflows, decision-making, and innovation.
Whether in engineering, business, healthcare, or the arts, the ability to work with AI tools responsibly and effectively will be critical for success in a rapidly evolving workforce.
These competencies aim to prepare students for both the opportunities and challenges AI presents in their future careers and broader society.
To help students achieve these standards, NJIT is offering a free non-credit 20 hour AI Literacy microcredential - expected to be launched during the Spring 2025 semester.
- AI Literacy and Fundamentals
- Explain key AI concepts such as machine learning, natural language processing, and generative AI, and identify their relevance in different fields.
- Critically evaluate misinformation or misunderstanding about the capability or application of AI and related technologies and/or identify an instance in which AI was used to spread misinformation.
- Demonstrate real-world applications of AI, including its impact on industries such as healthcare, business, engineering, and the arts.
- Machine Learning Awareness
- Differentiate between various machine learning (ML) types (e.g., supervised, unsupervised, and reinforcement learning) and explain how they apply to solve different problems in different disciplines
- Identify appropriate AI applications to solve a domain-specific problem, demonstrating their practical uses in tasks like prediction, content generation, or data analysis.
- AI in Context: History and Interdisciplinary Perspectives
- Articulate how AI has transformed problem-solving methodologies across disciplines, emphasizing the role of computational thinking in devising solutions that leverage AI technologies. Discuss how different fields apply AI to redefine problem scenarios, predict outcomes, and optimize processes, integrating AI into their unique disciplinary frameworks.
- Analyze the impact of AI across multiple disciplines (e.g., medicine, arts, economics, education) and evaluate how AI has transformed these fields regarding innovation, efficiency, and human experience.
- Examine how AI has been influenced by fields such as computer science, cognitive science, philosophy, linguistics, and ethics. Analyze how different disciplines have contributed to the development of AI technologies and their current applications.
- Describe the major milestones in AI development, from early computing to generative AI, and explain how key breakthroughs in AI have influenced industries, research, and society.
- Understanding Neural Networks and Deep Learning
- Explain the basic principles of neural networks and demonstrate their role in enabling AI systems to perform tasks such as pattern recognition and decision-making.
- Discuss the concepts of data scalability in AI by explaining the importance of large datasets in training AI models and how scaling data impacts the functionality and accuracy of these models.
- Describe the process of training and tailoring AI models; outline how AI models are generally trained on broad data sets and can subsequently be customized for specific purposes, emphasizing the adaptability of AI technologies.
- Assess the implications of data needs and scalability by evaluating how the requirement for extensive data influences issues such as privacy, bias, and the practicality of AI applications across various sectors.
- Prompting for Generative AI
- Formulate precise, context-aware prompts to generate high-quality responses (text, image, or other) from generative AI tools (e.g., ChatGPT, DALL·E).
- Revise and optimize prompts based on initial outputs to improve clarity, creativity, and relevance of AI-generated content.
- Demonstrate the use of prompt engineering across tasks such as content creation, problem-solving, and research within different disciplines.
- Critical Evaluation of Generative AI Outputs
- Assess the quality, reliability, and credibility of AI-generated content, identifying hallucinations, misinformation, and limitations.
- Evaluate the linguistic/rhetorical features of AI output (especially machine-generated text).
- Verify AI outputs by cross-referencing with credible sources and applying independent judgment to ensure accuracy and identify bias.
- Justify when and how generative AI tools should be used to assist learning while maintaining intellectual integrity.
- Ethical and Responsible Use of AI
- Evaluate the ethical concerns surrounding AI, including bias, privacy, environmental impact, intellectual property, copyright, and academic integrity, and apply responsible practices in academic and professional settings.
- Introduce explainable AI (XAI); explain the importance of making AI systems more transparent and understandable, especially those involving complex models that typically operate as "black boxes." Discuss the efforts and technologies aimed at making AI decisions more interpretable to users, which is crucial for building trust and accountability.
- Explore methodologies for testing AI transparency and fairness. Discuss how explainable AI can contribute to more ethical problem-solving, particularly in fields where decision-making needs to be justifiable.
- Analyze the societal impacts of AI on job markets, privacy, and inequality, and propose solutions or strategies for mitigating these issues while emphasizing the role of explainable AI in mitigating potential harms and ensuring equitable benefits.
- Evaluate AI tools for potential ethical risks and recommend best practices for ensuring fairness, transparency, and accountability in AI use.
- AI and Data Proficiency
- Assess the types and quantities of data needed for different AI solutions, identifying appropriate data scales for specific AI tasks.
- Select and justify AI methods based on data scale, detailing their advantages and limitations for small versus large datasets, and explaining their suitability for specific projects.
- Analyze datasets used in AI models to identify potential biases and evaluate data quality, considering the impact on model accuracy and fairness.
- Develop and apply strategies for ethical data collection and use in AI applications, ensuring compliance with privacy laws and ethical standards.
- Adaptability and Lifelong Learning with AI
- Engage in continuous learning to keep pace with advancements in AI technologies and practices.
- Collaborate with AI systems and human colleagues in interdisciplinary environments, balancing technological skills with critical thinking, empathy, and creativity.
- Utilize AI systems to help organize tasks and foster personal growth.
- Responsible and Innovative Use of AI in Academics
- Use AI Ethically: Apply principles of academic integrity when using AI (if permitted) and identify your institution’s policies or guidelines for student use of AI, ensuring that your contributions are genuine and citing AI assistance appropriately to avoid misrepresentation.
- Leverage AI for Learning Enhancement: Identify opportunities to use AI for brainstorming, structuring projects, or generating study aids, fostering innovation and growth rather than short cutting the learning process.
- Balance AI Use with Personal Development: Set personal goals for using AI in ways that enhance your skills and thinking, recognizing when to rely on AI tools and when to engage independently.